
Early work considered simple, coarse-grained features derived from mouse cursor data to be surrogate measurements of user interest ( Goecks and Shavlik, 2000 Claypool et al., 2001 Shapira et al., 2006). Inferring Interestįor a long time, commercial search engines have been interested in how users interact with Search Engine Result Pages (SERPs), to anticipate better placement and allocation of ads in sponsored search or to optimize the content layout. These analyses highlight potential use cases of our dataset, thereby allowing researchers to investigate similar environments and behaviors. In what follows, we provide a brief survey of what others have accomplished by analyzing mouse cursor movements in web search tasks. In practice, commercial web search engines often use mouse cursor tracking to improve search results ( Huang et al., 2011, 2012), optimize page design ( Leiva, 2012 Diaz et al., 2013), and offer better recommendations to their users ( Speicher et al., 2013). Researchers have started to analyze mouse movements on websites for the detection of neurodegenerative disorders ( White et al., 2018 Gajos et al., 2020). Mouse biometrics is another active research area that has shown promise in controlled settings ( Lu et al., 2017 Krátky and Chudá, 2018). Several works have investigated closely the utility of mouse cursor data in web search ( Arapakis et al., 2015 Lagun and Agichtein, 2015 Liu et al., 2015 Arapakis and Leiva, 2016 Chen et al., 2017) and web page usability evaluation ( Arroyo et al., 2006 Atterer et al., 2006 Leiva, 2011b), two of the most prominent use cases of this technology. Others have also noted the utility of mouse cursor analysis as a low-cost and scalable proxy of eye tracking ( Huang et al., 2012 Navalpakkam et al., 2013). It has been argued that mouse movements can reveal subtle patterns like reading ( Hauger et al., 2011) or hesitation ( Martín-Albo et al., 2016), and can help the user regain context after an interruption ( Leiva, 2011a). Mueller and Lockerd (2001) investigated the use of mouse tracking to create compelling visualizations and model the users' interests. (2001) were among the first ones to note a relationship between gaze position and cursor position during web browsing. Research in mouse cursor tracking has a long track record. Our dataset has associated attention labels and five demographics attributes that may help researchers to conduct several analysis, like the ones we discuss later in this section. We have collected mouse cursor tracking logs from near 3K subjects performing a transactional search task that together account for roughly 2 h worth of interaction data. For example, previous work has evidenced that when the mouse cursor is motionless, the user is processing information ( Hauger et al., 2011 Huang et al., 2011 Diriye et al., 2012 Boi et al., 2016), i.e., essentially “users first focus and then execute actions” ( Martín-Albo et al., 2016). and thus having access to this kind of data can lead to an overall better understanding of the user's cognitive processes. A user click is often preceded by several valuable interactions, such as scrolling, hovers, aimed movements, etc. However, click data provide an incomplete picture of user interaction, as they inform mainly about a users' end choice. With every click, digital footprints are created and logged, providing a detailed record of a person's online activity. Objective measurements of attentional processes are increasingly sought after by researchers, advertisers, and other key stakeholders from both academia and industry. Attention is a finite resource, so people spend their time on things they find valuable, especially when browsing online.
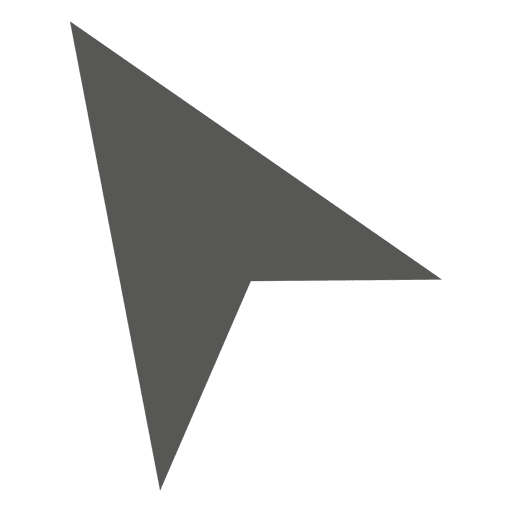

We introduce a large-scale dataset of mouse cursor movements that can be used to predict user attention, infer demographics information, and analyze fine-grained movements.
